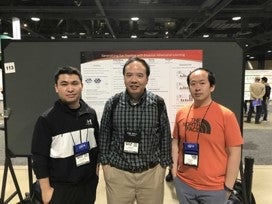
CVPR is the premier annual computer vision event comprising the main conference and several co-located workshops and short courses. With its high quality and low cost, it provides an exceptional value for students, academics and industry researchers. CVPR 2019 is hosted in Long Beach, CA. 3 papers came from Cognitive and Immersive Systems Lab (CISL) - the research collaboration between the IBM Research and Rensselaer Polytechnic Institute. CISL is one research center in the AI Horizon Network (AIHN) university collaboration program.
The three papers published by CISL focus on deep learning and computer vision based human computer interaction technologies. They are as follows:
1. Neuro-inspired Eye Tracking with Eye Movement Dynamics, by Kang Wang, Hui Su, Qiang Ji
Generalizing eye tracking to new subjects/environments remains challenging for existing appearance-based methods. To address this issue, we propose to leverage on eye movement dynamics inspired by neurological studies. Studies show that there exist several common eye movement types, independent of viewing contents and subjects, such as fixation, saccade, and smooth pursuits. Incorporating generic eye movement dynamics can therefore improve the generalization capabilities. In particular, we propose a novel Dynamic Gaze Transition Network (DGTN) to capture the underlying eye movement dynamics and serve as the topdown gaze prior. Combined with the bottom-up gaze measurements from the deep convolutional neural network, our method achieves better performance for both within-dataset and cross-dataset evaluations compared to state-of-the-art. In addition, a new DynamicGaze dataset is also constructed to study eye movement dynamics and eye gaze estimation.
2. Generalizing Eye Tracking with Bayesian Adversarial Learning, by Kang Wang, Rui Zhao, Hui Su, Qiang Ji
Existing appearance-based gaze estimation approaches with CNN have poor generalization performance. By systematically studying this issue, we identify three major factors: 1) appearance variations; 2) head pose variations and 3) over-fitting issues with point estimation. To improve the generalization performance, we propose to incorporate adversarial learning and Bayesian inference into a unified framework. In particular, we first add an adversarial component into traditional CNN-based gaze estimator so that we can learn features that are gaze-responsive but can generalize to appearance and pose variations. To further improve the generalization, we extend the point-estimation based deterministic model to a Bayesian framework so that gaze estimation can be performed using all parameters instead of only one set of parameters. Besides improved performance on several benchmark datasets, the proposed Bayesian adversarial learning also enables online adapting the model to new subjects/environments, demonstrating the potential usage for practical real-time eye tracking applications.
3. Bayesian Hierarchical Dynamic Model for Human Action Recognition, by Rui Zhao, Kang Wang, Hui Su, Qiang Ji
Human action recognition remains as a challenging task partially due to the presence of large variations in the execution of action. To address this issue, we propose a probabilistic model called Hierarchical Dynamic Model (HDM). Leveraging on Bayesian framework, the model parameters are allowed to vary across different sequences of data, which increase the capacity of the model to adapt to intraclass variations on both spatial and temporal extent of actions. Meanwhile, the generative learning process allows the model to preserve distinctive dynamic pattern for each action class. Through Bayesian inference, we are able to quantify the uncertainty of the classification, providing insight during the decision process. Compared to state-ofthe-art methods, our method not only achieves competitive recognition performance within individual dataset but also shows better generalization capability across different datasets. Experiments conducted on data with missing values also show the robustness of the proposed method.